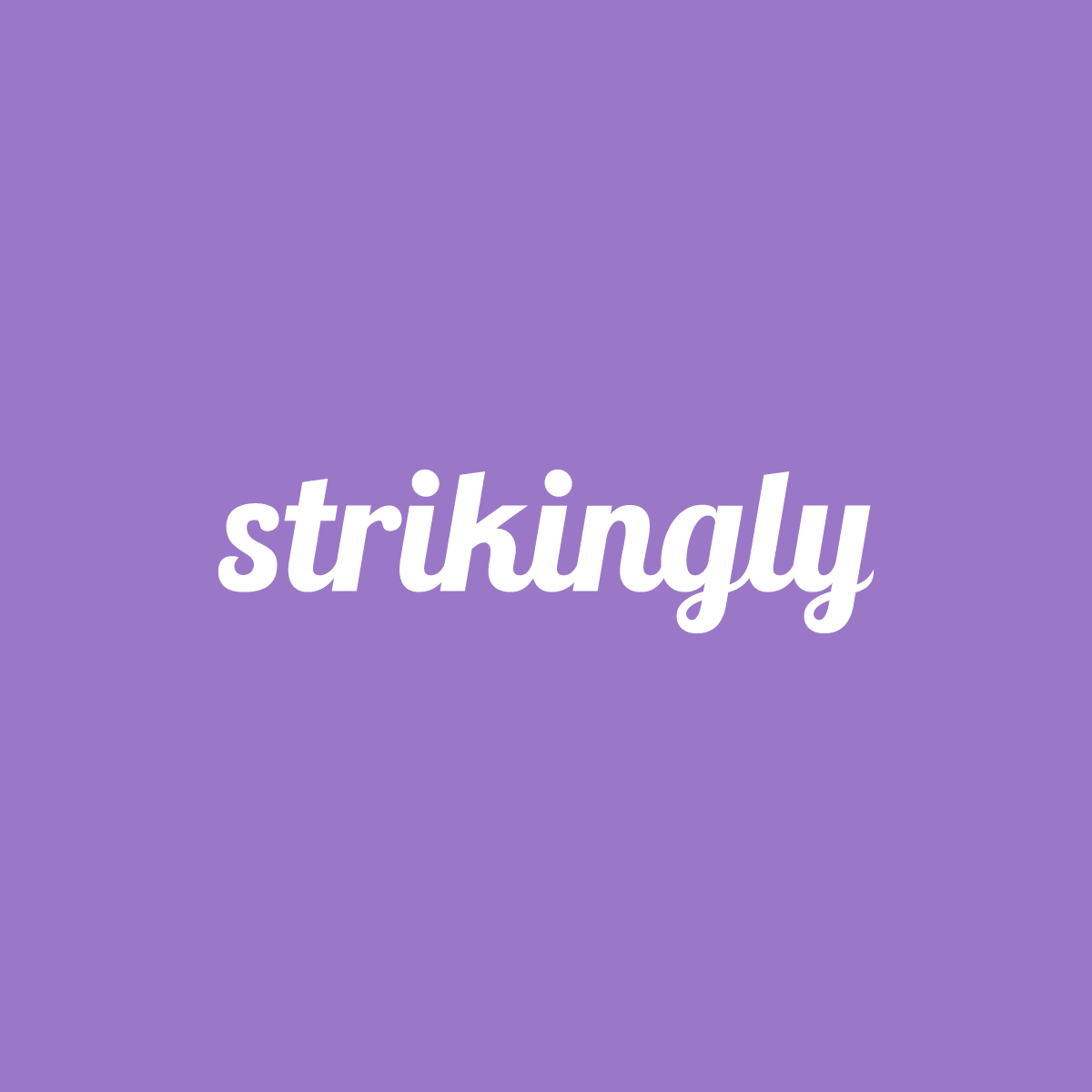
Unmixing before Fusion: A Generalized Paradigm for Multi-Source-based Hyperspectral Image Synthesis
Yang Yu*, Erting Pan*, Xinya Wang, Yuheng Wu, Xiaoguang Mei†, Jiayi Ma
Wuhan University
Highlights
- Formulate a generalized paradigm for multi-source based hyperspectral image (HSI) synthesis, incorporating a series of deep generative models.
- Pioneer to synthesis abundance (low-dimensional) instead of HSl sample (high-dimensional).
- Incorporate multi-source data to aleviate the issue of limited sample availability.
Brief Review
Comparisons of existing HSI synthesis. (a)The physical-modeling method induces the HSI formed by the bidirectional reflectance distribution function (BRDF). (b) Affine transformation applies the corresponding transformation to the original HSI. (c) Spectral super-resolution performs spectral expansion to obtain the HSI of the same scene as RGB. (d) Our proposed method can generate new HSI samples by multi-source fusion.
Framework
Synthesis Examples
Objective
Generating a vast quantity of diverse, reliable, and high fidelity synthetic HSl samples.
Key oberservation
Similar scenes share common low-rank features that can be described by a few endmembers, while the differences between scenes can be captured in their abundance maps.
Pipeline
Step 1: Unmixing across multi-source data
Step 2: Fusion-based synthesis
Examples of synthetic HSIs of remote sensing scenes
Examples of synthetic HSIs of nature scenes
Typical examples of synthesis abundances, generated HSIs, and corresponding spectral profile
Illustration on each band of the generated synthetic HSIs in the remote sensing scenario, and the last one is the corresponding false-color image (based on bands 15, 32, 49).
Code and Citation
https://github.com/HSI-Synthesis/Unmixing-before-fusion
Yu Y, Pan E, Wang X, et al. Unmixing Before Fusion: A Generalized Paradigm for Multi-Source-based Hyperspectral Image Synthesis[C]//Proceedings of the IEEE/CVF Conference on Computer Vision and Pattern Recognition. 2024: 9297-9306.MLA